Met Éireann is delighted to showcase some of its programmes in support of Ireland’s overarching theme for our participation in Expo 2020: “Island of Inspiration – putting creativity at the centre of human experience in the 21st century”.
Climate Change & Biodiversity
Establishing a Flood Forecast Centre
In the winter of 2015/2016, devastating floods, the result of record rainfall and a sequence of active storms which swept across Ireland, led to damage to the road network alone estimated at over €100m. Subsequently, the Irish Government decided to develop a National Flood Forecast and Warning Service (NFFWS) in order to better protect its citizens from flooding events, in the context of a warming world in particular.
The operational element of the National Flood Forecast and Warning Service, the Flood Forecast Centre (FFC), is being developed and will be implemented by Met Éireann, the Irish Meteorological Service. The FFC will initially perform a flood watch for fluvial (river) and coastal flooding for Ireland at both national and catchment level. The Office of Public Works (OPW) will provide guidance and standards.
Before the establishment of the Flood Forecast Centre, there had never been a national fluvial flood forecast model. There were a number of local flood forecast systems such as for the Lee and Suir rivers, but nothing at national level. The OPW were running a non-operational Tide and Storm Surge Forecast (TSSF) service model which was used to predict storm surges. However, as the OPW are not an operational organisation it was not used as such.
Met Éireann initially identified the optimal fluvial flood forecast model for Ireland at both national and catchment level. This consisted of a literature review of fluvial models and the formulation of a multi-criteria analysis of all of the models, including the inclusion of an integrator system. After a period of initial testing where a number of fluvial models were trialed in two different integrator systems, the FFC selected the HYPE model from the Swedish Meteorological Hydrological Institute (SMHI) and the FEWS Integrator System from Deltares.
Attention was then turned to the TSSF coastal model and it is now being currently tested. Met Éireann successfully built and trialed the model on the European Centre for Medium-Range Weather Forecasts (ECMWF)’s High Performance Computing (HPC) Facility. This sped up the performance of the model and it also allowed the model to be used with more computational power. Further testing on the performance of the model and its suitability in an operational Flood Forecast Centre is ongoing.
The final element to the jigsaw will be the development of a cloud-based web portal that will visualise flood and weather observations and forecasts and will disseminate flood statements, alerts and advisories to key stakeholders in flood emergency management in Ireland. Met Éireann is expecting to trial the FFC from Q4 2021 to early Q2 2022.
Developing Climate Services – TRANSLATE
Climate services help decision-makers better manage risks and plan for opportunities arising from climate change. These services are based on scientifically robust information and connect the latest climate science with real-world solutions for specific sectors, such as water, transport, energy.
Met Éireann, along with its partners, has been helping to develop climate projections for Ireland for some time. In 2003, the Community Climate Change Consortium for Ireland (C4I) was established in Met Éireann to produce the first regional climate projections for Ireland. Met Éireann is now a partner in the international EC-Earth global climate modelling consortium working with leading experts from 12 European countries to develop and run state-of-the-art, Earth system-based climate projections and works with partners to downscale these global simulations to produce high-resolution climate projections for Ireland.
In 2021, Met Éireann launched a project entitled TRANSLATE aimed at standardising national climate projections for Ireland and developing climate services. In addition to assimilating the lessons learned from past and ongoing climate services research, the project’s objectives are:
- To standardise climate projections for Ireland;
- To document & prioritise sector specific climate service requirements;
- To develop sector specific climate services;
- To develop climate service communication strategy and material.
Met Éireann is teaming up with climate researchers from NUI Galway – Irish Centre for High-End Computing (ICHEC) and University College Cork – SFI Research Centre for Energy, Climate and Marine (MaREI) to deliver the TRANSLATE project. The team also consists of climate experts from University College Dublin as well as international expertise from the Dutch company Deltares and is steered by experts from the Department of Environment, Climate and Communications (DECC), the Environmental Protection Agency (EPA), Maynooth University – The Irish Climate Analysis and Research UnitS (ICARUS), the Marine Institute (MI), the Climate Action Regional Office (CARO) and the Met Office.
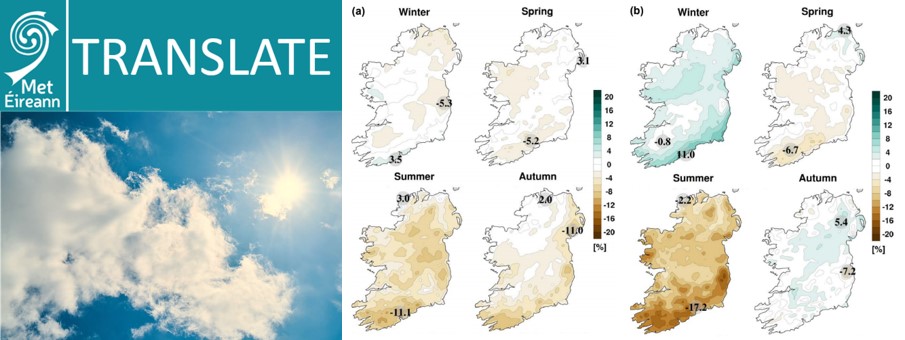
Figure 2: (left) TRANSLATE Research call (right) Mid-century seasonal projections of mean precipitation (%) for the (a) RCP4.5 and (b) RCP8.5 scenarios. In each case, the future period, 2041–2060, is compared with the past period, 1981–2000. The numbers included on each plot are the minimum and maximum projected changes, displayed at their locations.
Knowledge & Learning
Applying Artificial Intelligence and Machine Learning in Meteorology
On the 21st of September 2021, Met Éireann hosted the 2nd edition of its virtual Data Science Seminar. This seminar serves to showcase the work Met Éireann is currently undertaking in Artificial Intelligence / Machine Learning applications in meteorology.
Met Éireann’s Artificial Intelligence / Machine Learning work started with its partnership with the Centre for Research Training in the Foundations of Data Science in 2019. This programme is funded by SFI and seeks to train world class data science PhD graduates. As part of the PhD programme, the PhD candidates spend a 12-week internship period with an industry partner. Met Éireann has so far welcomed 7 PhD students since the beginning of the internships in 2020. These data scientists have worked on applying the power of Machine Learning to enhance Met Éireann’s numerical weather prediction (NWP) capabilities and complement its meteorological observational network, amongst other applications of this exciting and emerging technology.
Speakers at the 2021 edition of the seminar detailed work they undertook with Met Éireann on various topics including vegetation indexes in Met Éireann’s NWP model HARMONIE-AROME, analysis of weather information on Twitter, land cover maps for Ireland and predicting soil moisture at locations around Ireland.
Guest speakers from Maynooth University and the University of Exeter (UK) covered applications of Machine Learning in analysing satellite data and social sensing of social media posts during high-impact weather events. The event attracted over 90 participants with attendees from across Irish academia, semi-state bodies such as Teagasc and Enterprise Ireland and European meteorological services.
The 2020 edition of the Met Éireann Data Science seminar led to 2 scientific publications on applications of Machine Learning in NWP.
The first of these illustrates how Machine Learning can help produce a very high-resolution land-cover map for Ireland. Land-cover maps in NWP models are vital for having correct descriptions of land types (such as forests, lakes, hedgerows, cities etc.). This work uses satellite data and Machine Learning algorithms to improve how these land cover types are classified. A sample of the results from this project are outlined in Figure 1, illustrating how Machine Learning leads to enhanced land-cover maps.
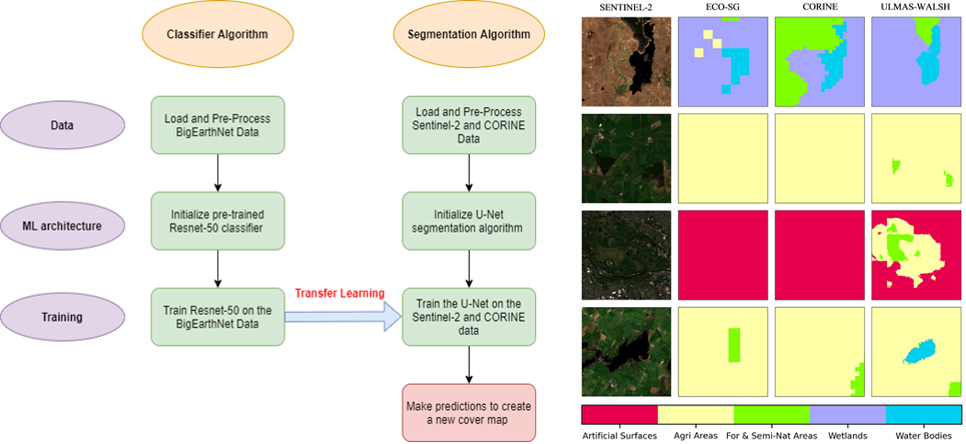
Figure 1: Workflow for application of Machine Learning algorithms (left image) and results of application to land-cover maps in databases in use at Met Éireann (right image). The Machine Learning map, entitled “Ulmas-Walsh”, clearly outperforms the others (ECO-SG, CORINE) compared to the satellite image (SENTINEL-2).
The second of these scientific publications demonstrated the use of Machine Learning in the improving precipitation forecasts from Ensemble Prediction Systems (EPSs). EPSs are the main forecasting tool in use by Met Éireann’s forecasters for predicting high-impact weather events, therefore it is imperative to make correct use of their output. This work employs Machine Learning algorithms to process EPS output in order to improve how they forecast significant and impactful precipitation events. A sample of the results are given in Figure 2 and demonstrate that approaches which use Machine Learning techniques improve the precipitation forecasts more significantly than other techniques.
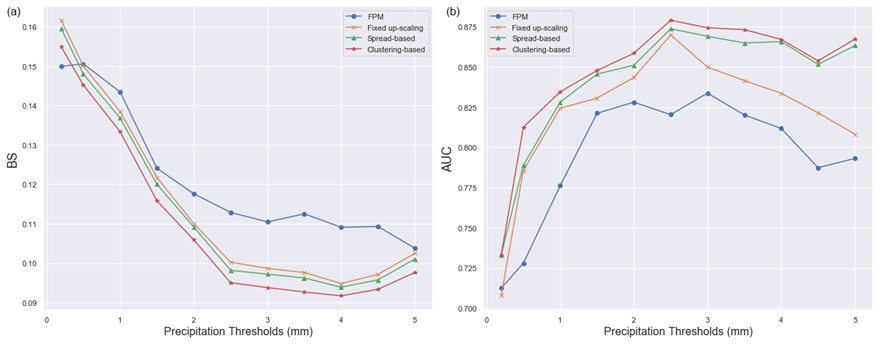
Figure 2: Verification of how various techniques improve Met Éireann’s forecasts of precipitation. For image (a), when the line is closer to the bottom of the graph, this indicates superiority, and the opposite is true for image (b). The red line dominates in both images, corresponding to the improvements from using a Machine Learning approach.